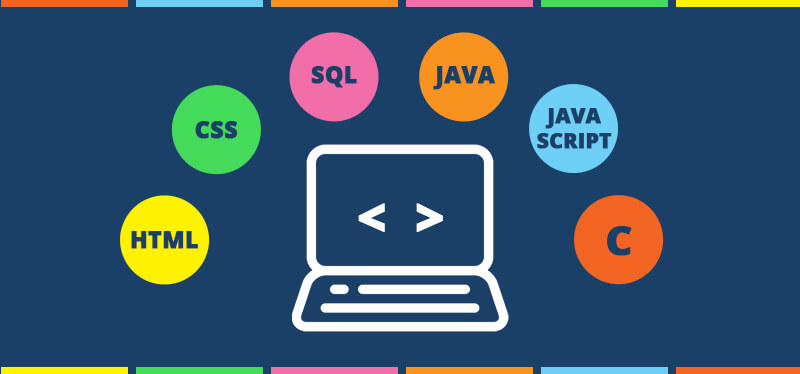
- By Faiza Mumtaz 27-Jun-2023
- 438
Computational models are powerful tools designed to confront the complex pollution footprint of plastic in the environment. By simulating the behavior, fate, and impacts of plastics, these models enable scientists and policymakers to understand the sources and distribution of plastic pollution, evaluate its ecological and human health effects, and develop targeted mitigation strategies. Through data integration, scenario analysis, and predictive capabilities, computational models play a crucial role in guiding efforts to reduce plastic pollution and protect the environment.
Introduction to the Plastic Pollution Problem
Plastic pollution has become a pressing global issue, with the excessive production and improper disposal of plastic causing severe environmental and health consequences. Plastics, due to their durability and widespread use, have infiltrated ecosystems worldwide, contaminating oceans, rivers, and land. This pollution poses significant threats to marine life, as marine animals mistakenly ingest or become entangled in plastic debris. Additionally, microplastics, small plastic particles that result from the breakdown of larger plastic items, have pervaded water bodies, impacting aquatic organisms and potentially entering the food chain.
Furthermore, plastic pollution has adverse effects on human health, as toxic chemicals from plastics can leach into the environment and accumulate in the food we consume. The complex nature of plastic pollution necessitates innovative computational models to understand its intricacies, assess its footprint, and develop effective mitigation strategies.
Understanding the Complexity of Plastic Pollution
Plastic pollution is a multifaceted issue that involves numerous interconnected factors, making it a complex challenge to address. Several key aspects contribute to the complexity of plastic pollution:
Diversity of Plastics: Plastics encompass a wide range of materials with varying chemical compositions, physical properties, and degradation rates. This diversity makes it difficult to generalize the behavior and impact of all plastics in the environment.
Ubiquitous Use and Disposal: Plastics are used in countless applications and industries, leading to their extensive production and consumption. Improper disposal practices, such as littering and inadequate waste management infrastructure, contribute to the accumulation of plastic waste in the environment.
Fragmentation and Persistence: Plastics do not biodegrade easily; instead, they break down into smaller fragments called microplastics. These microplastics can persist in the environment for extended periods, posing long-term risks to ecosystems and organisms.
Transport and Fate in the Environment: Plastic waste can be transported through various pathways, including rivers, wind, and ocean currents, leading to the dispersion of plastic pollution across vast distances. The fate of plastics in different environments is influenced by factors such as weathering, degradation, and interactions with organisms and sediment.
Ecological and Health Impacts: Plastic pollution affects diverse ecosystems, from terrestrial habitats to marine and freshwater environments. It can harm wildlife through ingestion, entanglement, or habitat degradation. Additionally, the potential transfer of plastic particles and associated toxins up the food chain poses risks to human health.
Socioeconomic and cultural factors: The issue of plastic pollution involves socioeconomic and cultural dimensions, including consumer behavior, industry practices, policy frameworks, and societal attitudes toward waste management and recycling.
Importance of Computational Models in addressing plastic pollution
Computational models play a vital role in addressing the complex and far-reaching issue of plastic pollution. They provide a valuable tool for scientists, policymakers, and environmental organizations to understand, assess, and develop effective strategies to mitigate plastic pollution. Here are some key reasons why computational models are important in addressing plastic pollution:
Prediction and Assessment: Computational models enable researchers to predict and assess the magnitude, sources, and distribution of plastic pollution in different environments. By simulating the behavior and fate of plastics, models can estimate the impact on ecosystems, wildlife, and human health. This information is crucial for developing targeted interventions and allocating resources effectively.
Scenario Analysis: Models allow for the exploration of different scenarios and the assessment of the potential outcomes of various interventions. For example, they can evaluate the effectiveness of different waste management strategies, recycling initiatives, or policy interventions in reducing plastic pollution. This helps decision-makers identify the most efficient and sustainable approaches.
Identifying Hotspots and Sources: Computational models can help identify hotspots of plastic pollution, both geographically and sector-wise. They can pinpoint areas where plastic waste accumulates the most, enabling targeted cleanup efforts and pollution prevention measures. Models can also identify specific industries or activities that contribute significantly to plastic pollution, guiding policy interventions and regulations.
Designing Mitigation Strategies: Models provide a platform to design and optimize mitigation strategies for plastic pollution. By simulating the outcomes of different interventions, such as plastic waste reduction, recycling initiatives, or alternatives to single-use plastics, models help identify the most effective and sustainable solutions. This can guide the development of policies, technologies, and behavior change campaigns.
Data Integration and Knowledge Synthesis: Computational models facilitate the integration of diverse data sources, including field observations, laboratory experiments, and remote sensing data, to create a comprehensive understanding of plastic pollution. They help synthesize existing knowledge, identify data gaps, and guide future research directions.
Cost-effectiveness and Scalability: Models offer a cost-effective and scalable approach to assess and address plastic pollution. They can simulate large-scale scenarios and provide insights that would otherwise be challenging or costly to obtain through field studies alone. This scalability allows for broader assessments and the evaluation of long-term trends and impacts.
Types of Computational Models for Assessing Plastic Pollution
Several types of computational models are used to assess and understand the impacts of plastic pollution. These models utilize various approaches and methodologies to simulate the behavior, fate, and effects of plastics in different environmental systems. Here are some key types of computational models used for assessing plastic pollution:
Material Flow Analysis (MFA) Models: MFA models track the flow of materials, including plastics, throughout their life cycle. These models quantify the inputs, outputs, and stocks of plastics at different stages, such as production, use, and disposal. MFA models help identify the major sources and sinks of plastic waste, estimate recycling rates, and assess the effectiveness of waste management strategies.
Life Cycle Assessment (LCA) Models: LCA models assess the environmental impacts of plastics throughout their entire life cycle, from the extraction of raw materials to end-of-life disposal. These models consider factors such as energy consumption, greenhouse gas emissions, and resource depletion associated with different stages of plastic production, use, and disposal. LCA models help evaluate the sustainability of plastic materials and guide the development of eco-friendly alternatives.
Environmental Fate and Transport Models: These models simulate the movement and fate of plastics in various environmental compartments, such as oceans, rivers, and sediments. They consider factors like ocean currents, winds, and hydrodynamics to predict the distribution and accumulation of plastic debris. Environmental fate and transport models provide insights into the spatial patterns and hotspots of plastic pollution, aiding in cleanup efforts and pollution prevention.
Ecological Risk Assessment Models: These models assess the potential impacts of plastic pollution on ecosystems and organisms. They integrate data on plastic exposure levels, toxicity, and ecological responses to evaluate the risks posed by plastics to different species and habitats. Ecological risk assessment models can help prioritize areas or species at higher risk and inform management strategies for protecting biodiversity.
Microplastic Modeling: Microplastic models focus specifically on the behavior and fate of microplastic particles in aquatic environments. They simulate the processes of fragmentation, transport, and deposition of microplastics, taking into account factors such as particle size, settling rates, and interactions with sediments and biota. Microplastic models contribute to understanding the distribution and ecological impacts of these tiny plastic particles.
Oceanic Circulation Models: These models simulate ocean currents and dynamics to understand the transport and accumulation of plastic debris in marine environments. By integrating physical and biological data, oceanic circulation models can provide insights into the pathways and destinations of plastics, as well as their interactions with marine ecosystems. They are particularly useful in predicting the movement of large-scale plastic pollution, such as oceanic garbage patches.
Challenges in Developing Accurate Computational Models for Plastic Pollution
Developing accurate computational models for plastic pollution is challenging due to the multifaceted nature of the problem and the inherent complexities associated with plastic waste dynamics. These challenges arise from the need to incorporate factors such as diverse plastic types, varying degradation rates, complex environmental processes, and the influence of human behavior and societal factors.
Additionally, the scarcity of comprehensive and high-quality data on plastic pollution further hinders the accuracy and reliability of models. Overcoming these challenges requires interdisciplinary collaboration, improved data collection efforts, refinement of model parameterization, and a deeper understanding of the interactions between plastic pollution and environmental systems.
Conclusion
In conclusion, computational models are indispensable tools for understanding and addressing the complex challenges of plastic pollution. Despite facing challenges such as data limitations, variability in plastic behavior, and the dynamic nature of plastic waste management systems, these models provide valuable insights into plastic pollution sources, behavior, and impacts. By refining and improving these models, we can enhance our understanding of plastic pollution and develop effective strategies to mitigate its environmental and health consequences.