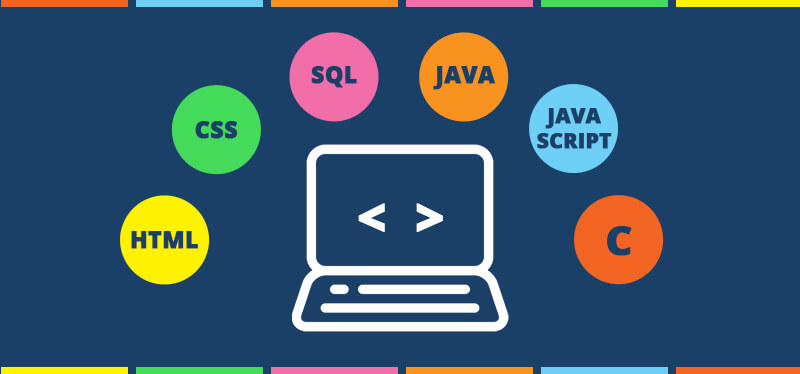
- By Shoaib Khan 21-Nov-2023
- 112
Title: The Evolutionary Wave: The Impact of Artificial Intelligence on Software Testing
Introduction: In the ever-evolving landscape of software development, the integration of artificial intelligence (AI) is reshaping traditional paradigms. One of the profound areas witnessing a transformative shift is software testing. This article explores how AI is revolutionizing software testing methodologies, unlocking new dimensions of efficiency, accuracy, and agility.
I. AI-Powered Test Automation: Redefining Speed and Accuracy Traditionally, test automation has been a cornerstone of efficient testing processes. However, the infusion of AI takes automation to unprecedented levels. Machine learning algorithms enable dynamic test script generation, self-healing test suites, and intelligent maintenance, reducing the burden on testing teams and accelerating time-to-market.
II. Predictive Analysis for Efficient Test Case Prioritization AI's prowess in predictive analysis is a game-changer for test case prioritization. By analyzing historical data, AI algorithms can predict which test cases are more likely to discover defects, allowing testing teams to focus on critical areas. This proactive approach enhances test coverage and ensures that resources are allocated where they are needed most.
III. Shift-Left Testing: Enhancing Collaboration Between Development and Testing AI facilitates the implementation of shift-left testing, where testing is integrated earlier into the development lifecycle. By providing instant feedback on code changes, AI-driven tools empower developers to catch and fix defects early, reducing the likelihood of issues cascading into later stages. This collaborative approach fosters a culture of quality throughout the development process.
IV. Cognitive Testing: Mimicking Human-like Testing Scenarios Cognitive testing, powered by AI, brings a human-like touch to testing scenarios. Natural language processing (NLP) and computer vision enable systems to comprehend and interact with applications in ways that simulate user experiences. This ensures that software is not only functionally sound but also user-friendly and intuitive.
V. Intelligent Test Data Management: Enhancing Test Coverage AI contributes to intelligent test data management by generating diverse and realistic datasets. Test scenarios that mimic real-world conditions are crucial for comprehensive testing. AI algorithms can create synthetic data, ensuring that the testing process covers a wide range of scenarios, including edge cases and unusual inputs.
VI. Adaptive Test Planning: Responding to Changing Requirements In a dynamic development environment, requirements can change rapidly. AI assists in adaptive test planning by analyzing changing project parameters and automatically adjusting testing strategies. This flexibility ensures that testing efforts remain aligned with evolving project goals and timelines.
VII. The Role of AI in Security Testing: Unmasking Vulnerabilities Security testing, a critical aspect of software quality assurance, benefits significantly from AI capabilities. Machine learning algorithms can identify patterns indicative of security vulnerabilities, detect anomalies, and proactively fortify software against potential cyber threats.
Conclusion: As the symbiotic relationship between AI and software testing continues to strengthen, the industry is witnessing a paradigm shift in how quality assurance is approached. The integration of AI is not merely a technological upgrade; it's a transformative force that empowers testing teams to deliver software faster, more reliably, and with enhanced quality. Embracing AI in software testing isn't just an option for the future—it's the key to staying ahead in the ever-evolving realm of software development.
Jack Carter
2 hours agoi think that some how, we learn who we really are and then live with that decision, great post!
you can view the more detail via link https://www.youtube.com/watch?v=HpZgwHU1GcIChing xang
2 hours agoi think that some how, we learn who we really are and then live with that decision, great post!
Danial Comb
2 hours agoi think that some how, we learn who we really are and then live with that decision, great post!
Jack Carter
2 hours agoi think that some how, we learn who we really are and then live with that decision, great post!